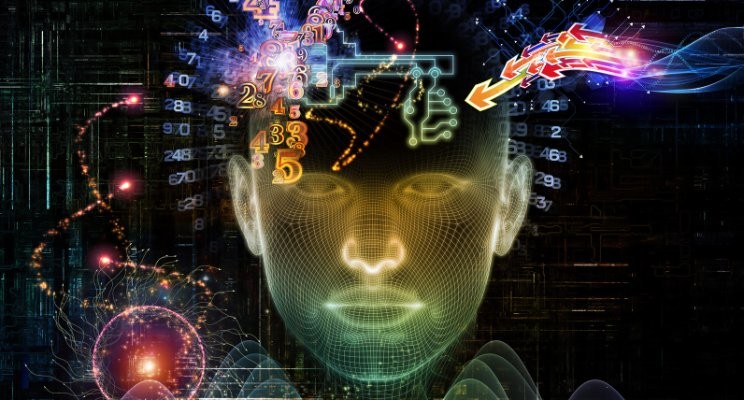
Intelligence Predictive Analysis to Reduce Production Cost
Postdoctoral Research Fellow at the University of Queensland and Data Scientist at Mining3.
This article addresses the problem of estimating the Rolling Resistance (RR) in surface mines. The project is motivated by the enormous costs associated with haulage, typically 50% of mine operation costs, and the knowledge that the differential structure of these expenses is closely linked to the interaction between tyre and road. The hauling productivity in a surface mine is dependant on a haul truck’s speed. In turn, the speed of a haul truck is affected by road quality. A prime indicator of haul road quality is rolling resistance.
RR is defined as the sum of all external forces acting on the road surface and opposite to the direction of the moving haul truck. RR is caused by the friction force between the tyre and the road surface (See Figure 1). RR as a part of total resistance plays a critical role in the productivity, fuel consumption, gas emissions, maintenance and safety of haul truck operations in surface mines.
Figure 1: Rolling Resistance and the most influential parameters
RR can measure by some different methods. RR measurement can be made under laboratory conditions, generally on “test drum surface”. This is a testing rig consisting of the tyre to be tested and a drum with a different outer surface which can rotate, simulation the movement of the tyre over a road surface. Some sophisticated mathematical methods can then be applied to the values of Drum Torque, Power and Tyre Force measured during testing to determine the RR experienced by the tyre.
Measurement of RR can also be obtained for a specific mine haul roads using on-site testing. This method uses a specially designed trailer towed behind a truck measure the force between the truck and trailer, used to pull it across the road surface. They also measure the grade of the haul road and acceleration. This data is then used with the relevant mathematical expression to determine RR of the haul road.
All currently mentioned methods to measure the RR are very expensive and inaccurate. An investigation about using advanced data analytics as an innovative approach to predict the rolling resistance on large off-high way trucks used by the mining sector has been conducted by a group of data scientists from Mining3. Mining3 is an industry-driven, global leader in mining research and innovation located in Brisbane, Australia.
Some parameters are affecting RR, which can be categorised into four groups. These groups are Road, Tyre, System and Weather condition. Identification of all effective parameters on RR and finding a correlation between these parameters and RR is very complex. That is because they are not independent parameters and they have an effect on each other as well.
To get information on the RR value of some haul road segments in the real condition in mine site, the use of VIMS[1] is critical. VIMS can provide information for the Total Resistance(TR) value of the road surface. Total resistance is defined as the sum of Grade Resistance (GR)and RR. GR is the resistance force caused by the grade of haul road; it is equal to the gradient of the haul road in percent (See Figure 2).
Figure 2: Grade Resistance (GR)
In this project, a forward Artificial Intelligence Model (AIM) was developed to find the correlation between Haul Truck Fuel Consumption (FC), Truck Speed (S), Payload (P) and RR (See Figure 3). This computer model was trained and tested by some collected data sets from a large copper mine located in the north of Arizona, USA. This mine represents one of the largest copper reserves in the United States and the world. The deposit of this mine was estimated reserves (in 2015) of 907 million metric tonnes of ore grading 0.26% copper and 0.03% molybdenum.
Figure 3: Rolling Resistance Predictive Model
Simulation of RR was completed by developing a backwards AIM (See Figure 3). This model could predict the RR based on the haul truck fuel consumption, truck speed and payload. It means that developed model can be potentially an RR estimation tool resulting tyre life prediction and maintenance cost reduction.
References:
1. Soofastaei A, Aminossadati SM, Arefi MM, Kizil MS. Development of a multi-layer perceptron artificial neural network model to determine haul trucks energy consumption. International Journal of Mining Science and Technology. 2016;26(2):285-93.
2. Soofastaei A, Aminossadati SM, Kizil MS, Knights P. A Discrete-Event Model to simulate the effect of payload variance on truck bunching, cycle time and hauled mine materials. International Journal of Mining Technology. 2016;2(3):167-81.
[1] VIMS: Vital Information Management System
Responses